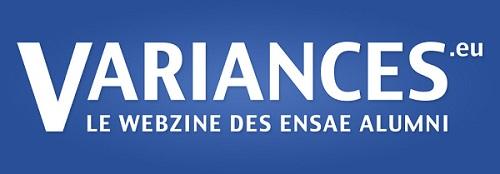
Liste des articles
In a landmark achievement for artificial intelligence, a poker bot developed by researchers in Canada and the Czech Republic has defeated several professional players in one-on-one games of no-limit Texas hold’em poker.
Perhaps most interestingly, the academics behind the work say their program overcame its human opponents by using an approximation approach that they compare to “gut feeling.”
“If correct, this is indeed a significant advance in game-playing AI,” says Michael Wellman, a professor at the University of Michigan who specializes in game theory and AI. “First, it achieves a major milestone (beating poker professionals) in a game of prominent interest. Second, it brings together several novel ideas, which together support an exciting approach for imperfect-information games.”
Later this week, a tournament at a Pittsburgh casino will see several world-class poker players play the same version of poker against a program developed at CMU. Tuomas Sandholm, a professor of computer science at CMU who is leading the effort, says the human players involved are considerably stronger than those tested by the Alberta researchers, and 120,000 hands will be played over 20 days, providing greater statistical significance to the results. The tournament could confirm that AI has indeed mastered a game that has long seemed far too complex and subtle for computers.
DeepStack, the poker-playing software that has already bested some professional players, was developed by a team led by Michael Bowling, a professor of computer science at the University of Alberta, which included researchers from Charles University and Czech Technical University in the Czech Republic. In a research paper posted online but not yet peer reviewed, the researchers say that DeepStack played almost 45,000 hands of poker against several players, beating them handily.
Poker is more complex than many other games that have pitted humans against AI. And tellingly, it contains levels of uncertainty, such as when an opponent may be bluffing, that are found in many real-world situations that AI has not yet mastered. Poker players cannot see their opponents’ hands, meaning that, in contrast to checkers, chess, or Go, not all of the information contained within the game is available to them. Researchers from DeepMind, a U.K.-based subsidiary of Alphabet, made headlines last year after creating a program capable of beating one of the world’s best Go players (see “Google’s AI Masters the Game of Go a Decade Earlier Than Expected”).
Heads-up no-limit Texas hold’em is a version of the game played between two people who can bet as many of the chips as they possess. This variant for a long time proved too difficult for machines to play expertly. There are 10160 (10 followed by 160 zeros) possible paths of play for each hand in heads-up no-limit Texas hold’em.
DeepStack learned to play poker by playing hands against itself. After each game, it revisits and refines its strategy, resulting in a more optimized approach. Due to the complexity of no-limit poker, this approach normally involves practicing with a more limited version of the game. The DeepStack team coped with this complexity by applying a fast approximation technique that they refined by feeding previous poker situations into a deep-learning algorithm.
“What's really new for such a complex game is being able to effectively compute the action to take in each situation as it is encountered, rather than having to work through a simplified form of the entire tree of game possibilities offline,” says Wellman of the University of Michigan.
The researchers compare DeepStack’s approximation technique to a human player’s instinct for when an opponent is bluffing or holding a winning hand, although the machine has to base its assessment on the opponent's betting patterns rather than his or her body language. “This estimate can be thought of as DeepStack’s intuition,” they write. “A gut feeling of the value of holding any possible private cards in any possible poker situation.”
It’s possible to measure the performance of a poker player by looking at the amount won, relative to the amount bet at his or her table, over many games. DeepStack had a win rate roughly nine times better than what would be considered good for a professional player.
In 2015, Bowling and colleagues at the University of Alberta “solved” the more limited version of heats up hold’em by developing a poker bot capable of playing the game perfectly.
The poker bot involved in the Pittsburgh tournament, called Libratus, was developed by Sandholm and one of his graduate students, Noam Brown. The pair has not yet disclosed details of how their program approaches the game, but Brown says it essentially tries to "solve" the game—or figure out every possible scenario—earlier during the game than was previously possible. Libratus runs on extremely powerful hardware at the Pittsburgh Supercomputing Center, a facility run jointly by CMU and the University of Pittsburgh.
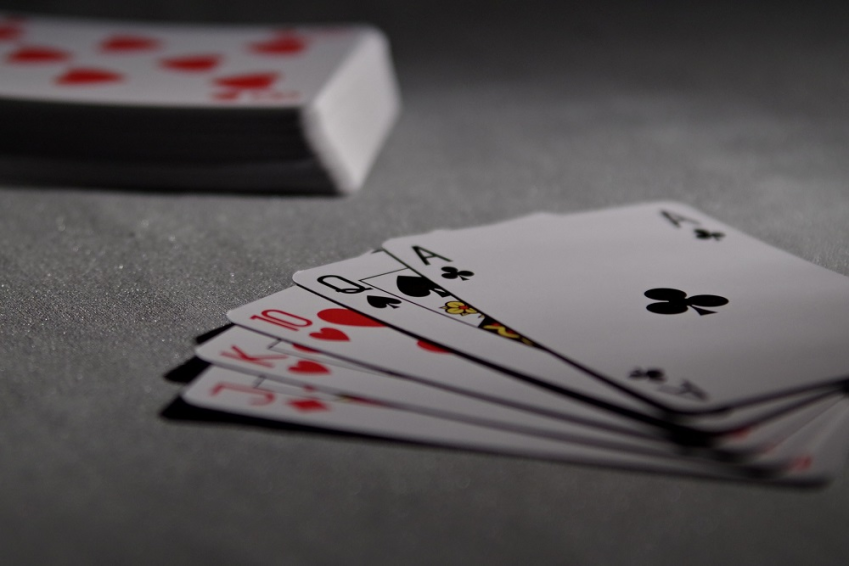
Vue 160 fois
23 janvier 2017
Poker is the latest game to fold against artificial intelligence
Publié par
From US
| Machine Learning
Apres la défaite de professionnels du jeu de Go en 2016 face a DeepMind de Google, voici un recent article du MIT Technology review sur de nouvelles avancées de l’intelligence artificielle et la défaite de professionnels de Poker face a un nouveau programme DeepStack.
Un article sélectionné par Frédéric Smadja (2000), le correspondant de Variances.eu aux Etats-Unis : l'article, rédigé par Will Knight, a été précédemment publié dans le "MIT Technology review"
Two research groups have developed poker-playing AI programs that show how computers can out-hustle the best humans.
Perhaps most interestingly, the academics behind the work say their program overcame its human opponents by using an approximation approach that they compare to “gut feeling.”
“If correct, this is indeed a significant advance in game-playing AI,” says Michael Wellman, a professor at the University of Michigan who specializes in game theory and AI. “First, it achieves a major milestone (beating poker professionals) in a game of prominent interest. Second, it brings together several novel ideas, which together support an exciting approach for imperfect-information games.”
Later this week, a tournament at a Pittsburgh casino will see several world-class poker players play the same version of poker against a program developed at CMU. Tuomas Sandholm, a professor of computer science at CMU who is leading the effort, says the human players involved are considerably stronger than those tested by the Alberta researchers, and 120,000 hands will be played over 20 days, providing greater statistical significance to the results. The tournament could confirm that AI has indeed mastered a game that has long seemed far too complex and subtle for computers.
DeepStack, the poker-playing software that has already bested some professional players, was developed by a team led by Michael Bowling, a professor of computer science at the University of Alberta, which included researchers from Charles University and Czech Technical University in the Czech Republic. In a research paper posted online but not yet peer reviewed, the researchers say that DeepStack played almost 45,000 hands of poker against several players, beating them handily.
Poker is more complex than many other games that have pitted humans against AI. And tellingly, it contains levels of uncertainty, such as when an opponent may be bluffing, that are found in many real-world situations that AI has not yet mastered. Poker players cannot see their opponents’ hands, meaning that, in contrast to checkers, chess, or Go, not all of the information contained within the game is available to them. Researchers from DeepMind, a U.K.-based subsidiary of Alphabet, made headlines last year after creating a program capable of beating one of the world’s best Go players (see “Google’s AI Masters the Game of Go a Decade Earlier Than Expected”).
Heads-up no-limit Texas hold’em is a version of the game played between two people who can bet as many of the chips as they possess. This variant for a long time proved too difficult for machines to play expertly. There are 10160 (10 followed by 160 zeros) possible paths of play for each hand in heads-up no-limit Texas hold’em.
DeepStack learned to play poker by playing hands against itself. After each game, it revisits and refines its strategy, resulting in a more optimized approach. Due to the complexity of no-limit poker, this approach normally involves practicing with a more limited version of the game. The DeepStack team coped with this complexity by applying a fast approximation technique that they refined by feeding previous poker situations into a deep-learning algorithm.
“What's really new for such a complex game is being able to effectively compute the action to take in each situation as it is encountered, rather than having to work through a simplified form of the entire tree of game possibilities offline,” says Wellman of the University of Michigan.
The researchers compare DeepStack’s approximation technique to a human player’s instinct for when an opponent is bluffing or holding a winning hand, although the machine has to base its assessment on the opponent's betting patterns rather than his or her body language. “This estimate can be thought of as DeepStack’s intuition,” they write. “A gut feeling of the value of holding any possible private cards in any possible poker situation.”
It’s possible to measure the performance of a poker player by looking at the amount won, relative to the amount bet at his or her table, over many games. DeepStack had a win rate roughly nine times better than what would be considered good for a professional player.
In 2015, Bowling and colleagues at the University of Alberta “solved” the more limited version of heats up hold’em by developing a poker bot capable of playing the game perfectly.
The poker bot involved in the Pittsburgh tournament, called Libratus, was developed by Sandholm and one of his graduate students, Noam Brown. The pair has not yet disclosed details of how their program approaches the game, but Brown says it essentially tries to "solve" the game—or figure out every possible scenario—earlier during the game than was previously possible. Libratus runs on extremely powerful hardware at the Pittsburgh Supercomputing Center, a facility run jointly by CMU and the University of Pittsburgh.
Aucun commentaire
Vous devez être connecté pour laisser un commentaire. Connectez-vous.